The Automatic Ingestion Monitor (AIM) was developed and validated in our previous and ongoing NIH-funded projects. The AIM is a passive food intake sensor requiring no self-report of eating episodes, just compliance with wearing the device. The AIM (AIM-2) capable of automatic detection of food intake, characterization of meal microstructure and capturing images of the food being eaten.
1. Food intake detection and image capture by Automatic Ingestion Monitor.
There are several versions of the AIM sensor that are described in published research1–6. The version of AIM (AIM-2) to be used in this project is a clip-on to eyeglasses (with non-corrective or corrective lens) containing a still 5Megapixel camera aligned with person’s gaze (Fig. 1). The food intake is detected by the built-in accelerometer7 and a non-contact optical sensor. The optical sensor monitors the temporalis muscle and allows for precise chew count during food intake4,5. The data from the accelerometer and optical sensors are processed in real time on the device. Detected food intake triggers the camera that takes periodic images (every 5 to 15 seconds) of the food being eaten (Fig. 2). The sensor data and images are stored on the SD card with the capacity up to 4 weeks of data. A full charge of AIM’s battery (housed in the enclosure, Fig.1) allows for 20 hours of non-stop image capture or 2-3 days of meal time capture.
AIM achieved 96% accuracy (F1-score) of food intake detection in the community by monitoring the activity of temporalis muscle. The time resolution of food intake detection was 3 seconds. The sensor was able to estimate chew counts with an average mean absolute error of 3.8%. The images of the food can be annotated using standard nutritional databases (Fig. 3). Only sensor-detected episodes need to be entered, greatly minimizing the effort.
2. Accurate measurement of meal microstructure.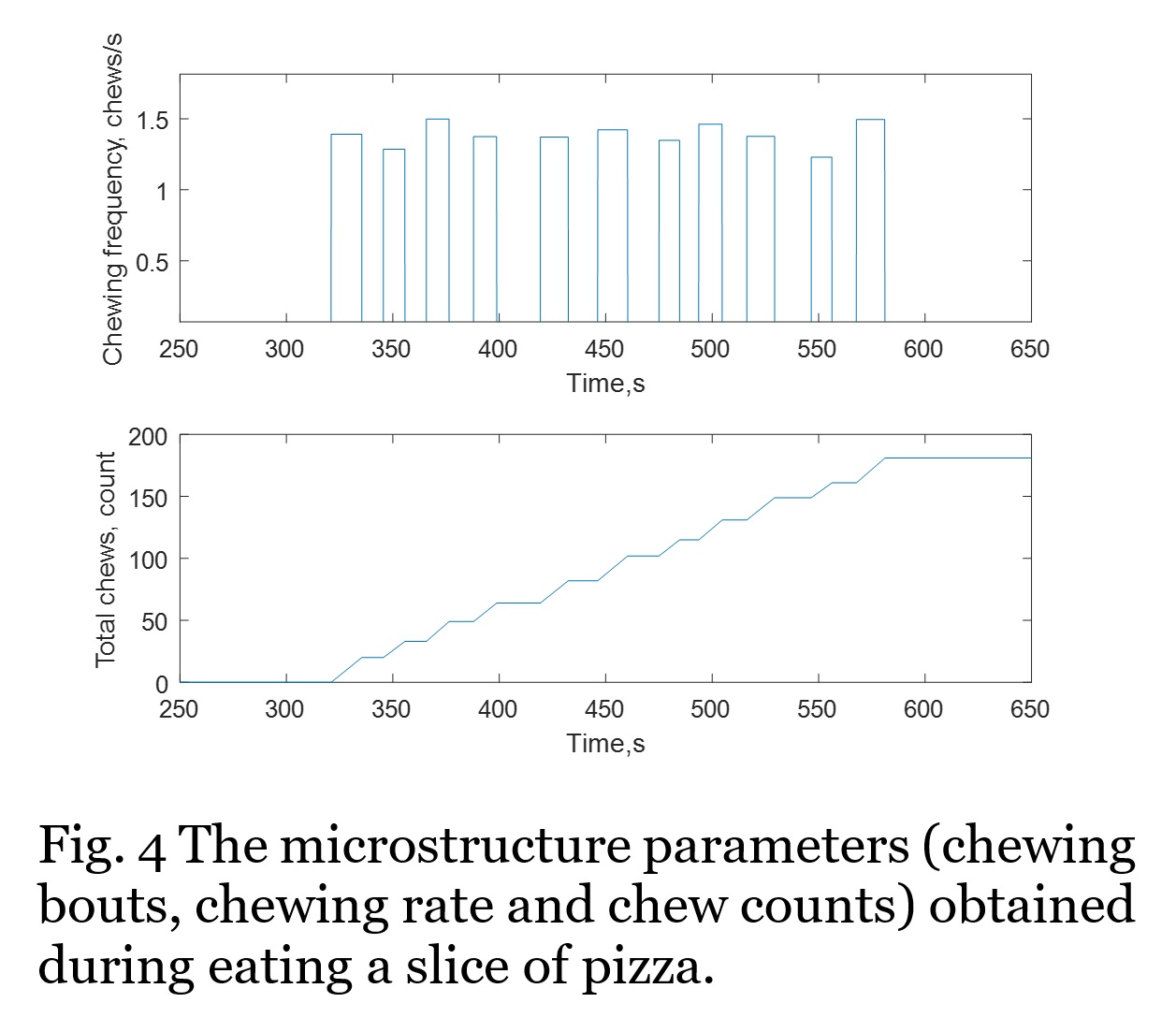
Meal microstructure includes factors such as eating episode duration (the duration from the start of the meal to the end including pauses), duration of actual ingestion (time spent eating in a given eating episode), the number of eating events (a bite, potentially followed by a sequence of chews and swallows), rate of ingestion, number of chews, chewing frequency, chewing efficiency and bite size. A wealth of preclinical data exists illustrating the importance of meal microstructure to caloric intake and weight control in animal modelsbut detailed human studies are rare. Among the few human studies that do exist, there are demonstrated differences in meal microstructure in obese versus lean people, men versus women, and among individuals in various states of health. The meal microstructure parameters provide quantifiable metrics of ingestive behavior that may be used to trigger feedback specific to certain behaviors.
In several recent studies5,8 we have demonstrated ability of the AIM to accurately measure the duration of eating episodes, duration of ingestion, number of eating events, number of chews and chewing rate (Fig. 4). We have also demonstrated that desired time resolution of sensor-based food intake detection should be ≤5s to accurately detect meal microstructure.
3. Demonstrated correlation of chew counts with the mass and energy content of a meal.
Chewing and swallowing are correlated with food intake in an obvious way. Chews, swallows and hand-to-mouth gestures can also serve as predictors of ingested mass and EI3,9–11. Since Counts of Chews and Swallows (CCS) do not directly measure caloric content of food, the approach to EI estimation by CCS is indirect and similar to the way most physical activity monitors indirectly estimate energy expenditure (most monitors do not directly measure heat production by the body but use dependent predictors such as acceleration, heart rate, etc.).
In our earlier work10 we compared estimation of the energy content of unrestricted meals from CCS to weighed food records and self-report. In a laboratory setting, 30 healthy participants consumed three meals of identical content (training meals) and a fourth meal with different content (validation meal). Participants chose the content of both meals and the manner and sequence of food intake was not restricted. EI for each meal was estimated by three different methods: weight of the amount consumed (as measured by research staff) coupled with energy per serving from the Nutrition Facts label, self-report, and CCS models. For the training meals, individually-calibrated CCS models showed significantly lower absolute assessment errors (15.8%±9.4%)_than self-report (27.9%±29.7%) when both were compared with the known amount (P < 0.05). CCS models were also not biased toward underreporting.
4. Ability to change eating behavior based on feedback from AIM.
In a recent work12 we explored the potential use of AIM for providing feedback on the progression of a meal and tested a JITAI aimed at reduction the total food mass intake in a single meal. Eighteen participants consumed three meals each in a lab while monitored by AIM that was tracking chew counts. The baseline visit was used to establish the self-determined ingested mass and the associated chew counts. Real-time feedback on chew counts was provided in the next two visits, during which the target chew count was either the same as that at baseline or the baseline chew count reduced by 25% (in randomized order). The target was concealed from the participant and from the experimenter. Nonparametric repeated-measures ANOVAs were performed to compare mass of intake, meal duration, and ratings of hunger, appetite, and thirst across three meals. JIT feedback targeting a 25% reduction in chew counts resulted in an average reduction of 10% in mass and energy intake without affecting perceived hunger or fullness.
5. Privacy protection in AIM-based studies.
Use of wearable cameras is a separate, stand-alone issue not specific to AIM. The expectation is that eyeglass-mounted cameras soon will be as ubiquitous as cameras on smartphones. Augmented-reality devices such as Google Glass, Apple AR glasses, Microsoft HoloLens, and Amazon’s Alexa frames are about to become mainstream. We successfully addressed the privacy issue in our studies in 3 ways. 1) The study procedures treat collected data according to an established ethical framework; 2) AIM device captures images only during food intake and does not capture images when food intake is not detected. In our studies this alleviated privacy concerns of users dramatically6, from concern value of 5 out of 7 (concerned) to concern value of 1.9 (not concerned)1. 3) In our recent publication13, we have reported on selective removal of HIPAA-protected information from images of food intake. Our method automatically removes faces, body parts, and all computer screens (Fig. 5). All three protective measures will be utilized as a part of the proposed work.
6. Compliance. The studies indicate excellent compliance with AIM wear. The AIM-2 has been successfully used or being used in studies (1 to 10 days in duration) of adults and children as young as 4y.o. in the USA6, UK14, Australia, and urban and rural Ghana and Uganda15 (Africa). The wearable device is well-tolerated and can be used both by regular eyeglass wearers and people who are not normally wearing the eyeglasses. The study provides a personally sized frame with non-corrective lenses for such individuals. In a recently completed study of 28 individuals wearing AIM-2 sensor for 2 days in the community6, we measured 12h 38m/day (±1h 27m STD) of mean use time (not being on the charger) and 10h 7m hours/day (±1h 41m STD) of mean wear time (worn as prescribed), equivalent to 80% compliance with wear, consistent with the provided instructions to take the device off during activities involving water (showering) or other private activities (bathroom use, etc.).
7. The capabilities of AIM are being continuously extended
- new sensors are introduced to extend capabilities;
- deep learning methods are used to automatically recognize food type and portion size, estimate energy intake;
- AIM sensors are used to estimate energy expenditure from physical activity;
- AIM-based Just-In-Time Adaptive Interventions are tested for ability to modify eating behavior.
8. Safety. AIM device is a non-significant risk device as it is not an implant, does not support life, does not present a health risk, and is not used in diagnosing or treating disease. AIM is based on low power, low-voltage (3V) electronics and poses no more than minimal risk, comparable to a consumer electronic device.
References
1. Sazonov, E. & Fontana, J. M. A Sensor System for Automatic Detection of Food Intake Through Non-Invasive Monitoring of Chewing. IEEE Sensors Journal 12, 1340–1348 (2012).
2. Fontana, J. M., Farooq, M. & Sazonov, E. Automatic Ingestion Monitor: A Novel Wearable Device for Monitoring of Ingestive Behavior. IEEE Transactions on Biomedical Engineering 61, 1772–1779 (2014).
3. Farooq, M. & Sazonov, E. A Novel Wearable Device for Food Intake and Physical Activity Recognition. Sensors 16, 1067 (2016).
4. Farooq, M. & Sazonov, E. Segmentation and Characterization of Chewing Bouts by Monitoring Temporalis Muscle Using Smart Glasses with Piezoelectric Sensor. IEEE Journal of Biomedical and Health Informatics PP, 1–1 (2016).
5. Farooq, M. & Sazonov, E. Automatic Measurement of Chew Count and Chewing Rate during Food Intake. Electronics 5, 62 (2016).
6. Doulah, A. B. M. S. U., Ghosh, T., Hossain, D., Imtiaz, M. H. & Sazonov, E. “Automatic Ingestion Monitor Version 2” — A Novel Wearable Device for Automatic Food Intake Detection and Passive Capture of Food Images. IEEE Journal of Biomedical and Health Informatics 1–1 (2020) doi:10.1109/JBHI.2020.2995473.
7. Farooq, M. & Sazonov, E. Accelerometer-Based Detection of Food Intake in Free-Living Individuals. IEEE Sensors Journal 18, 3752–3758 (2018).
8. Doulah, A. et al. Meal Microstructure Characterization from Sensor-Based Food Intake Detection. Front. Nutr. 4, (2017).
9. Sazonov, E. S. et al. Toward Objective Monitoring of Ingestive Behavior in Free-living Population. Obesity 17, 1971–1975 (2009).
10. Fontana, J. M. et al. Energy intake estimation from counts of chews and swallows. Appetite 85, 14–21 (2015).
11. Yang, X. et al. Estimation of mass and energy intake during a meal using information from bites, chews and swallows. in review (2017).
12. Farooq, M., McCrory, M. A. & Sazonov, E. Reduction of energy intake using just-in-time feedback from a wearable sensor system. Obesity (Silver Spring) 25, 676–681 (2017).
13. Hassan, M. A. & Sazonov, E. S. Selective Content Removal for Egocentric Wearable Camera in Nutritional Studies. IEEE Access (2020).
14. Jobarteh, M. L. et al. Development and Validation of an Objective, Passive Dietary Assessment Method for Estimating Food and Nutrient Intake in Households in Low- and Middle-Income Countries: A Study Protocol. Curr Dev Nutr 4, (2020).
15. McCrory, M. et al. Methodology for Objective, Passive, Image- and Sensor-based Assessment of Dietary Intake, Meal-timing, and Food-related Activity in Ghana and Kenya (P13-028-19). Curr Dev Nutr 3, (2019).